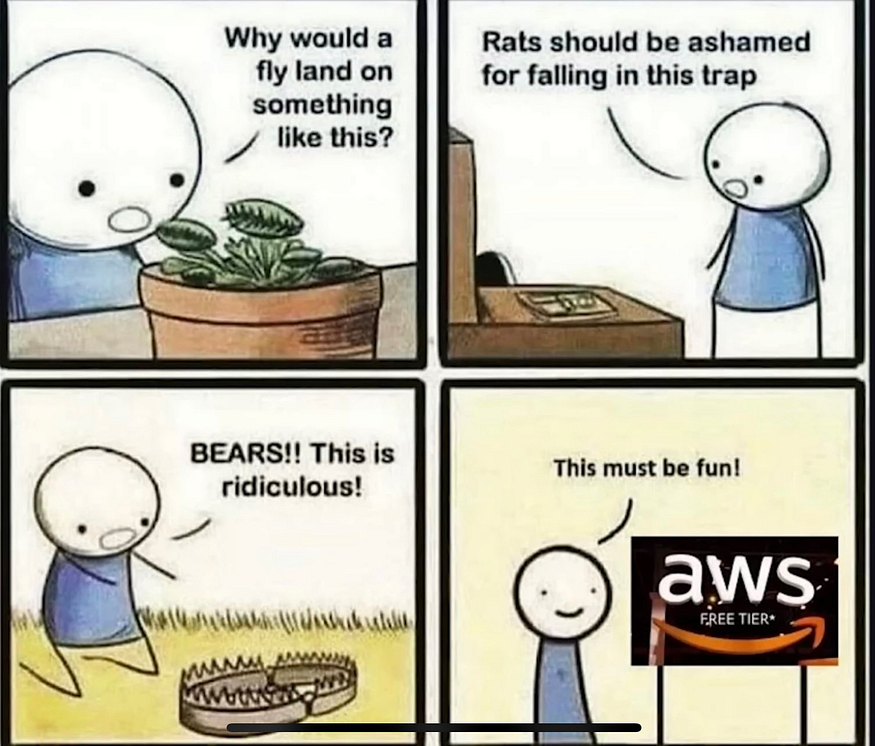
This AI newsletter is all you need #48
Last Updated on July 17, 2023 by Editorial Team
Author(s): Towards AI Editorial Team
Originally published on Towards AI.
What happened this week in AI by Louie
This week in AI has witnessed significant announcements from OpenAI as well as increasing pressure on the company, including Sam Altman’s testimony in the Senate, the release of the ChatGPT iOS app, and plugins for ChatGPT+ users.
During a Senate Committee hearing, OpenAI CEO Sam Altman, along with other industry leaders, largely agreed on the need to regulate the rapidly advancing AI technology developed by companies such as OpenAI, Google, and Microsoft. While we agree on the need for AI regulation, it has to be very carefully managed and executed and one key risk with more regulation is its potential to entrench the market leaders with high regulatory barriers to competition. For this reason some question the motives of large tech companies in calling for AI regulation. As a counter to this, in its “Governance of Superintelligence” OpenAI is advocating for the most burdensome regulation to only kick in over “a significant capability threshold”.
OpenAI was also busy with new releases including the “Continue generating” button in ChatGPT, aiming to enhance user experience. This function eliminates the need to manually input “continue” for longer responses and inputs, streamlining the process. In addition, OpenAI has recently launched the official ChatGPT iOS app and plans to release an Android version soon. The iOS app is free to use and incorporates a speech-to-text feature, enabling users to interact with ChatGPT through speech. Furthermore, OpenAI has introduced plugins exclusively for ChatGPT Plus subscribers, granting them access to web browsing and all the beta plugins. These beta plugins offer a diverse array of functionalities, including text-to-audio conversions and real-time data updates on stocks and cryptocurrencies.
As open-source AI models gain traction, and debate continues on the pros and cons of open source vs closed API models for AI going forward, OpenAI also announced the upcoming release of a new open-source large language model. Perhaps this comes due to increased pressure from the open-source LLM world. The specific details of OpenAI’s forthcoming open-source model have not been disclosed yet. The momentum of open-source AI also continued at Stability AI this week, which has made its DreamStudio platform open-source. The Stable Diffusion model that powers it has been open source from the start, but it is an interesting progression to see the commercial interface become open source also and a trend we will watch for more broadly.
– Louie Peters — Towards AI Co-founder and CEO
Hottest News
Stability AI is unveiling an open-source version of DreamStudio, a commercial interface designed for their AI image generator model called Stable Diffusion. This release empowers community developers to enhance and experiment with the interface, potentially leading to valuable improvements for the company.
2. Introducing the ChatGPT app for iOS
ChatGPT has launched an iOS app that includes open-source speech recognition for its subscribers. The app, available for free, allows users to sync their chat history across multiple devices. ChatGPT Plus subscribers get exclusive access to GPT-4’s capabilities, early access to features, and faster response times, all on iOS.
3. Meta bets big on AI with custom chips and a supercomputer
Meta has revealed its internal endeavors to develop AI workload infrastructure, specifically focusing on generative AI. They have introduced a new chip called the Meta Training and Inference Accelerator (MTIA). The MTIA is an ASIC that incorporates various circuits onto a single board, allowing it to be programmed to carry out one or many tasks in parallel.
4. Apple reveals new accessibility features, like custom text-to-speech voices
Apple previewed a suite of new features aimed at enhancing cognitive, vision, and speech accessibility. These tools are scheduled to be introduced on iPhone, iPad, and Mac devices later this year. Specifically designed for individuals with cognitive disabilities, Assistive Access is set to roll out across a range of Apple products in the coming year.
5. Zoom will soon integrate Anthropic’s chatbot across its platform
Zoom has announced a partnership with Anthropic to integrate the latter’s AI assistant into its productivity platform. The initial implementation of this integration will involve incorporating Claude into Zoom’s Contact Center product. The aim is to enhance customer support services by leveraging Claude’s capabilities to develop improved self-service features.
5-minute reads/videos to keep you learning
Anthropic’s 100k-token Claude model has ignited a debate regarding the necessity of document retrieval in question-answering (Q&A) systems. The retriever-less architecture performs well but has higher latency than retriever-based approaches, and is most suitable for small corpora and non-critical latency apps. This discussion raises important considerations regarding the trade-off between retrieval-based and retriever-less approaches in Q&A systems.
2. The “Three-Hills” Model for evaluating GPT startups
The “Three-Hills” Model provides an evaluation framework for assessing the success of GPT startups based on three key factors: “Productivity Enhancements,” “Non-zero-sum-game Value,” and “Moat = Value from Context.” These factors are assessed across three distinct levels. Additionally, the model identifies three categories of moats that can help prevent generic GPT displacement.
3. ChatGPT vs. Bard: A realistic comparison
The article conducts an unscientific field experiment to compare the performance of ChatGPT and Bard. The author subjects both models to various challenges, including summarizing lengthy content, composing a Python function, devising social media marketing tactics, and more.
4. A Decade In Data Engineering — What Has Changed?
Over ten years, numerous significant breakthroughs and tools emerged in the field of data engineering. It played a pivotal role in transforming the way data engineers operate. This article provides an overview of the history of data engineering, highlighting the key milestones that have shaped the field and brought us to the present state.
5. What is AI Art and How is it Created?
This article provides a comprehensive exploration of the concept of AI art, examining its meaning, the historical background of AI-generated art, the various types of AI employed in art generation, the ways artists utilize AI, the challenges involved in creating AI art, the ethical concerns associated with AI-generated art, and more.
Papers & Repositories
At Google, there was a document put together by Jeff Dean, the legendary engineer, called Numbers Every Engineer should know. Having a similar set of numbers for LLM (Language Model) developers is highly beneficial for quick estimations. This repository aims to share specific numbers utilized by Anyscale, explaining their significance and demonstrating how they can be leveraged to one’s advantage.
2. CodeT5+: Open Code for Large Language Models
Introducing CodeT5+: a collection of encoder-decoder LLMs designed for code, allowing flexible combinations of component modules to cater to diverse downstream code tasks. The analysis highlights the models’ state-of-the-art (SoTA) performance on various code-related tasks, including code generation and completion, math programming, and text-to-code retrieval.
3. Tree of Thoughts: Deliberate Problem Solving
The paper presents an innovative framework for language model inference called Tree of Thoughts (ToT). ToT builds upon the Chain of Thought approach commonly used to prompt language models but with the added capability of exploring coherent units of text referred to as “thoughts.” These thoughts serve as intermediate steps toward problem-solving.
4. mLongT5: A Multilingual and Efficient Text-To-Text Transformer for Longer Sequences
mLongT5 enhances the architecture of LongT5 by utilizing the multilingual datasets employed in pretraining mT5 and the pretraining tasks of UL2. The model underwent evaluation across several multilingual summarization and question-answering tasks, demonstrating superior performance compared to existing multilingual models like mBART or M-BERT.
5. Improving Language Model Negotiation with Self-Play and In-Context Learning from AI Feedback
This research delves into the possibility of utilizing different AI models, taking on the roles of buyer, seller, and critic in a negotiation game, to learn and enhance their deal-making abilities without human intervention. The study discovered that specific models were capable of learning from the game and improving their negotiation strategies.
Enjoy these papers and news summaries? Get a daily recap in your inbox!
The Learn AI Together Community section!
Weekly AI Podcast
In this week’s episode of the “What’s AI” podcast, Louis Bouchard interviews David Mertz, a senior developer, data scientist, and author. They delve into the fascinating world of generative AI and its applications in coding, including AI coding assistants like Copilot and ChatGPT. The discussion also addresses copyright concerns associated with generative AI and delves into the intricate issue of moral rights and attribution. David highlights the strengths and weaknesses of these assistants, elucidating how they can both assist and impede developers in their coding endeavors. You can find the podcast on YouTube, Spotify, or Apple Podcasts.
Meme of the week!
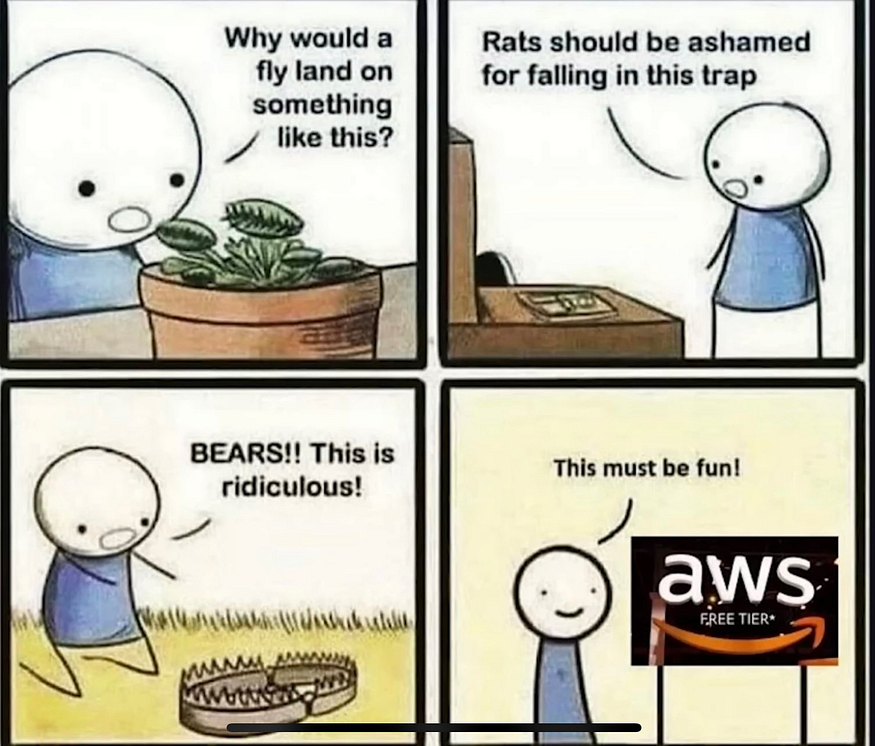
Meme shared by NEON#8052
Featured Community post from the Discord
Chris M#0847 recently introduced DataLab, an open-source Python implementation that is easy to use for all types of data, including images, text, tabular data, and audio. In Software 2.0, data has become the new code, models are the new compiler, and manual data validation is the new unit test. Datalab combines any ML model with innovative data quality algorithms to provide a linter for the Software 2.0 stack. It automatically analyzes datasets for “bugs” by adaptively checking for issues commonly found in real-world ML datasets, without requiring you to specify their potential form. Datalab’s checks consider all the relevant information learned by your trained ML model. Find it on GitHub or check the Jupyter tutorial here and support a fellow community member. Share your thoughts in the thread here!
AI poll of the week!

Join the discussion on Discord.
TAI Curated section
Article of the week
Compare ChatGPT to Machine Learning Techniques for Sentiment Analysis in 2023 by Courtlin Holt-Nguyen
Sentiment analysis, powered by NLP (Natural Language Processing), enables AI systems to comprehend the opinions and emotions expressed in text. The advanced NLP capabilities of ChatGPT have garnered significant attention in recent months. However, the question remains: Is ChatGPT superior to existing machine learning NLP techniques? This article aims to demonstrate ChatGPT’s notably enhanced accuracy compared to pre-ChatGPT NLP techniques.
Our must-read articles
Understanding LangChain U+1F99C️U+1F517: Part:2 by Chinmay Bhalerao
Building a Q&A Bot over Private Documents with OpenAI and LangChain by Sriram Parthasarathy
If you are interested in publishing with Towards AI, check our guidelines and sign up. We will publish your work to our network if it meets our editorial policies and standards.
Job offers
Data Processing Specialist @Hyperscience (Remote)
Senior Software Engineer — Backend @Harness (Mountain View, USA/ Hybrid)
Machine Learning Engineer @ConverseNow.ai ( Buenos Aires, Argentina/Hybrid)
Senior AI Data Trainer @Cohere (Remote)
Staff Machine Learning Engineer @Clari (Remote)
Senior Software Engineer (Python) @Reserv (Remote)
Software Engineering Manager @Lucidya (Remote)
Interested in sharing a job opportunity here? Contact [email protected].
If you are preparing your next machine learning interview, don’t hesitate to check out our leading interview preparation website, confetti!

Join thousands of data leaders on the AI newsletter. Join over 80,000 subscribers and keep up to date with the latest developments in AI. From research to projects and ideas. If you are building an AI startup, an AI-related product, or a service, we invite you to consider becoming a sponsor.
Published via Towards AI