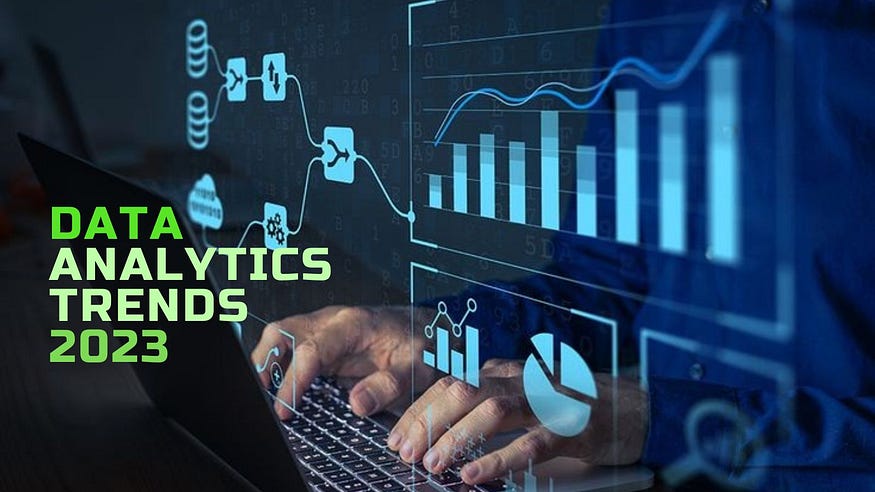
“Navigating the Data Odyssey: Top Data Analytics Trends for 2023”
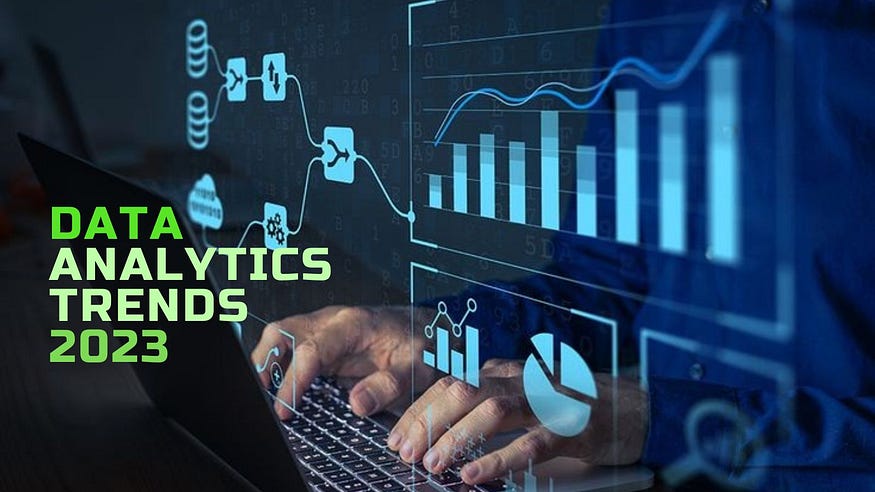
Last Updated on November 6, 2023 by Editorial Team
Author(s): Bhavesh Agone
Originally published on Towards AI.
The study of analyzing raw data to draw inferences about information is known as data analytics. In this blog, I’ll discuss the future of data analytics and show you where the sector is going. In recent years, this industry has experienced many significant changes. You must constantly keep up with the most recent developments in the industry and incorporate them into your work. Interestingly enough, several of these trends are already in motion, while some of these will take time, and others will depend on how far technology has advanced. But if you want to be a valuable addition to any organization, you must be aware of them. Well, it's very interesting- let’s dive in!!!

Artificial Intelligence
Let's start with Artificial intelligence. Data analysts and other professionals can be more productive when performing data analytics since data analytics is advancing alongside the development of AI. There are several fantastic new A.I. tools on the market right now that you can quickly integrate into your data analytics pipelines. The most effective AI augments rather than replaces human creativity and productivity. Why not have a look at Open AI’s Copilot, which is now integrated into GitHub and enables programmers to write code more effectively and quickly than ever before, if you want a coding-related example? GitHub Copilot is a pretty fantastic tool that greatly increases a programmer’s potential and productivity. Ask a programmer who has just tried it , the only response he could muster would be of amazement, awe, and wonder. Data analysts will, therefore, depend more on machine learning tools in 2023 and beyond to enable them to do their duties more effectively. Let’s look at some of the examples that are currently provided.
Smart Tooling: This refers to artificial intelligence (AI) programs that automatically transform data from unstructured formats, such as written documents, webpages, or Excel spreadsheets, into SQL-structured database systems. This eliminates a lot of the labor-intensive work that data analysts often perform in the early stages of a project.
Natural Language Processing: Natural language, instead of dated code bases like SQL or Python can now be used to query databases by data analysts. Natural language processing also gives analysts the ability to interactively monitor a specific company’s social media presence and keep track of things like brand awareness, popularity and sentiment analysis, etc. In structured data formats like knowledge graphs, analysts can include information about a company, such as its articles of incorporation or investor reports, so that it can be later more efficiently queried.
Time Series Analysis: Building machine learning models to automatically identify risk triggers in user-related data or financial data of the organization will enable more analytics based on such triggers. Many people are concerned that machines will eliminate their occupations when they learn about artificial intelligence.
Data Democratization
Giving all staff members access to data, whether or not they are technical professionals, is known as data democratization. Nowadays, everyone agrees that data-driven judgments result in superior choices. What is a choice driven by data? It entails depending on data rather than your gut feeling. As a result, an increasing number of businesses offer internal training to help staff members become more data literate.
Data literacy: What is it? It simply implies that each and every person of your staff needs to be knowledgeable about the methods and ideas involved in dealing with data.
However, just because data’s being democratized doesn’t mean we don’t need technical expertise. For example, technical expertise are still needed to supervise data analytics pipelines and to guide and assist non-technical staff members. The increased use of dashboarding technologies like Looker, Tableau, and Power BI is a good indicator of the democratization of data. However, departments outside of analytics are also making use of data. Data democratization is also necessary for departments like marketing, growth, and product, as evidenced by the emergence of tools supporting these divisions, such as AB Tasty for AB test, userpoll for guided onboarding, and Mixpanel or Heap for product analytics. The emergence of the API network is another area where data democratization is evident. Through the deployment of APIs, businesses are increasingly making their data publicly accessible.
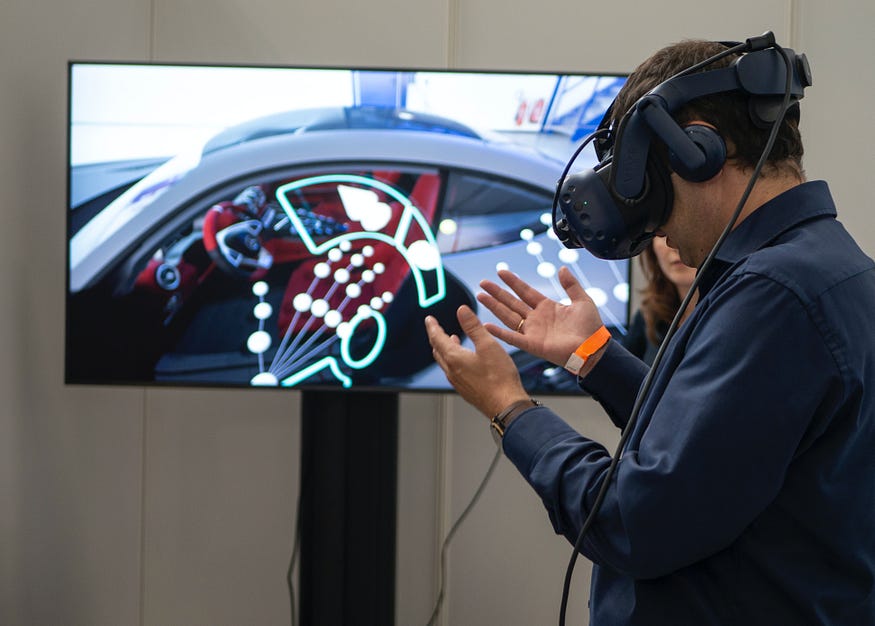
Embedded Analytics
Let’s now examine embedded analytics. Most likely, you’ve heard a lot about virtual reality (VR) and augmented reality (AR) in the news recently. Whether it’s the recent difficulties at Meta, the anticipated debut of Apple glasses in a few years, the new Oculus Quest Pro, or go back to the difficulties Google encountered when introducing Google Glass. Some of the buzz is, in fact, true. Analysts are now able to offer customers fresh ways to perceive and comprehend data thanks to developments in hardware for augmented reality and virtual reality. Users will be able to view more information than ever before about any goods and services they’re consuming thanks to embedded data that will be made available via AR Hardware. Imagine you’re in a grocery store and you can click a button to quickly learn a product’s carbon footprint, caloric content, exact source details, and any recipes you might be able to utilize it in. The eventual democratization of data is facilitated by this embedding of analytical information. Let’s now consider VR. We all realized the necessity of remote work during the most recent COVID outbreak. The lack of human connectedness caused by online meetings using services like Zoom and Google Meet is one of the major negatives of working remotely. So how does VR fit into that? With good avatars and realistic emotional expressions in completely immersive virtual boardrooms, it would be able to simulate the sense of connection that people experience when they are physically present in a setting close to one another. It enables the analysis of nonverbal signs like eye contact and others that are lost during these online interactions. Furthermore, consumers of data will be able to understand the messages that the analysts are attempting to convey more readily in a fully virtual environment where analysts can use data geometrically, interactively, and in three dimensions rather than via PowerPoint presentations or other more traditional techniques.Data analysts will have far more freedom to employ inventive methods to present their data in a fully virtual world. As a result, the adoption of VR will probably have the biggest impact on data visualization if it includes all the procedures that a data analyst needs, such as cleansing acquisition and preparation.

Predictive Analytics
The goal of predictive analytics is to make predictions about future events using historical data and analytics techniques like statistical modeling and machine learning. Any firm may now use historical and current data to accurately predict patterns and behaviors milliseconds, days, or years in the future with the aid of advanced predictive analytics tools and models. Predictive models are frequently used by retailers to plan for future inventory needs, coordinate shipment schedules, and optimize store layouts for sales. Predictive analysis tools will be used more frequently in the field of data analytics in the upcoming years.

Data as a Service (DaaS)
Data analytics won’t function the same way it does now in the future. Right now, if you want an efficient data analytics solution, you’ll often need to engage a few data engineers to build your data pipelines and a few data analysts to analyze the data within and produce efficient data visualizations to communicate with the rest of the organization. For many new enterprises, this cost may be prohibitive. Additionally, finding the necessary IT talent is not simple. And this is where DaaS can be of assistance. Data as a Service, or DaaS for short, is the name given to cloud-based software applications that let users manage their needs for data warehouses or business intelligence tools. These DaaS tools can be hosted and used from any location. They come in different sizes. Essentially, it enables subscribers to offer data analytics services without having to maintain a data engineering or data analytics department. Because you don’t need to hire as many data engineers, software engineers, and data Data analysts will have far more freedom to employ inventive methods to present their data in a fully virtual world. As a result, the adoption of VR will probably have the biggest impact on data visualization.DaaS should ultimately result in an increase in productivity for small and mid-sized businesses. As more companies integrate their products and services into the cloud, DaaS has become a more popular method for integrating, managing, and delivering data analytics services. It will enable analysts to share data more easily than before and will streamline business tasks and processes. Additionally, in recent years, a brand-new class of products — the so-called minimal code or no code analytics platforms — has grown in popularity. These are systems designed for non-programmers and typically feature drag-and-drop user interfaces. Without having any prior technical knowledge, users may develop analytics, pipelines, and visualization dashboards. This significantly lowers entry hurdles for small to midsize businesses trying to make data-driven decisions. yet without having enough money to pay for a sizable staff of internal analysts or engineers. Platforms for low- or no-code analytics include those from GoodData,Priceloop, and Bold BI.

Data Fabric
Data fabric is a comprehensive data and artificial intelligence approach to use all current and future investments in your data estate, not just one specific piece of technology. The AI Ladder is an easy-to-understand and implement strategy that focuses on gathering, analyzing, and incorporating all company data to provide better, more compelling customer experiences, better services, and better products, as well as increase business operation efficiency. So why is it so important to grasp what a data fabric is today? We’re entering a new technological era, so the biggest challenge in the knowledge era will be bridging the knowledge and insight gap so that more of the available data and information can be turned into knowledge and insights, resulting in more dynamic, cutting-edge products and services, more personalized customer experiences, and improved business operation efficiency. Therefore, what prevents organizations from filling in that gap with the data they already have? The basic answer is complexity, which is created by technology, scale, and human resources. To sum up, a data fabric is all about utilizing people and technology to bridge the knowledge gap between the company’s expertise and the data that is available, resulting in more dynamic goods and services, more compelling customer experiences, and increased business efficiency.
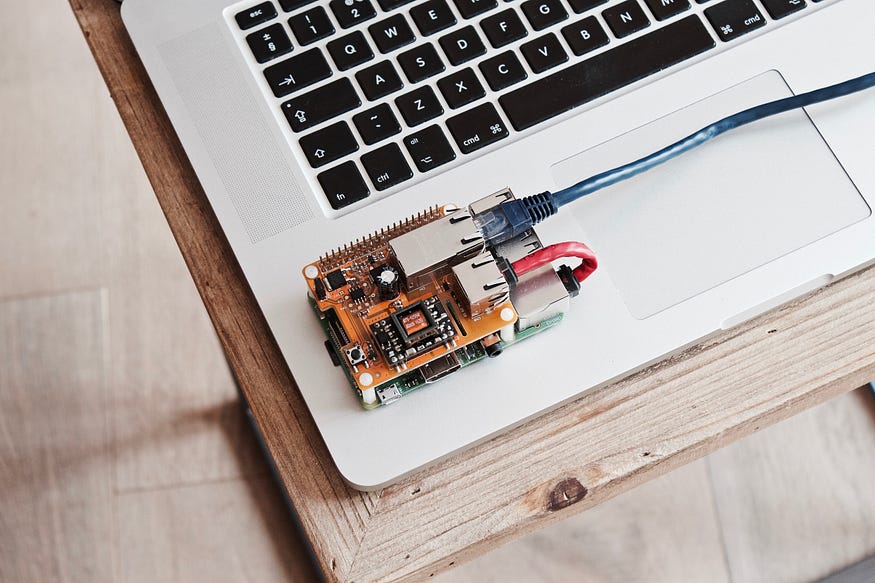
IoT and Real-time Data
The growth of IoT and real-time data comes next. Most of you have probably heard about the Internet of Things, or IoT, by this point. Whether it’s your corner smart speaker or your robotic vacuum. Hardware is increasingly utilized to monitor and study human behavior. More goods and services are producing real-time data as a result of the growth of IoT. Real-time data is frequently unstructured and large in volume. This implies that you receive a lot of it and that it is quite untidy. However, if you know how to work with unstructured, real-time data, you can discover priceless insights into the world that will enable you to add genuine economic value. One of the most recent examples is Ring, a smart device with an accompanying app that enables users to keep an eye on video and sound data throughout the house. IoT devices are also used in different industries and manufacturing facilities to monitor diffrent intrumenets and machines etc. Google Maps also makes use of IoT to track real-time traffic data and deliver alerts about clogged roads close to you.
Why, then, does this matter to data analysts? The more real-time data we can gather about a system’s users, the better our ability to comprehend them will be. Data analysts will thus be able to open up fresh opportunities for the companies they work for. Real-time data and the growth of IoT also raise questions about data protection. There you have it, then. The field of data analytics is one that changes quickly. You will always need to be alert in this field.
Data Governance
Let’s now examine data governance. As we are all aware, the modern economy generates and consumes an increasing amount of data. Concerns from individuals about how their data is being created and used are growing along with data governance. Data governance has become more popular recently as a result of these worries. And what exactly is data governance? Data governance refers to making sure that the data you produce is of the highest quality and that it complies with all applicable laws and regulations in the area in which it is both produced and used. Basically, let’s produce high-quality data while abiding by the law. Therefore, all businesses nowadays should take care to develop an effective data governance policy. A sound plan makes sure that data is protected and aims to make sure that it is as high-quality as feasible. Lack of a strategy can cause low-quality data to miss out on commercial possibilities and, in the worst scenario, result in penalties or even jail time from the authorities. Many of our followers in Europe will be familiar with GDPR regulations, but there are also equivalent laws in other regions of the world. So having sound data governance processes in place is crucial for all businesses. besides data governance techniques. To guarantee that the data is compliant with the data governance policy, small to medium businesses may frequently need to employ data protection officers. Companies are not the only ones who struggle with data governance, though. That responsibility falls on consumers as well. How recently have you truly read the terms and conditions of a contract on an app that you use or update? I always mention in my blogs that data has a great value in this world and companies would do anything to get it. I understand how difficult it is to read those terms & conditions, which is why legal technology companies are establishing themselves all over the world to assist us in overcoming the issues of data governance that we face as private data consumers. As a result, I see a market in the future for disruptive legal technology companies that use machine learning and artificial intelligence to help customers comprehend their contractual obligations. In this arena, though, there are already players.
CONCLUSION
So, here we conclude our list of the latest trends in the data analytics domain. It’s evident that this field is evolving rapidly. With cutting-edge technologies emerging, the growing utilization of data, and a stronger focus on data governance, the future of data analytics looks incredibly promising. To fully harness the potential of data in the coming years, it will be crucial to stay up to date with the trends in this field. So let's embark on this journey into a data-driven future with curiosity and creativity as we explore and gain insights, from the sea of data that surrounds us.
Please feel free to share your thoughts in the comment section. Your suggestions are always welcome.
Join thousands of data leaders on the AI newsletter. Join over 80,000 subscribers and keep up to date with the latest developments in AI. From research to projects and ideas. If you are building an AI startup, an AI-related product, or a service, we invite you to consider becoming a sponsor.
Published via Towards AI