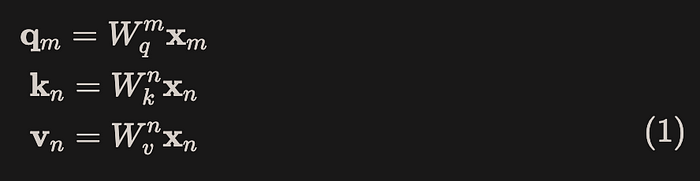
An In-depth exploration of Rotary Position Embedding (RoPE)
Last Updated on December 30, 2023 by Editorial Team
Author(s): Florian
Originally published on Towards AI.
including principles, visual illustrations, and code
Rotary Position Embedding (RoPE)[1] is a widely used positional encoding technique, which is utilized by many large language models such as Llama[2], Llama2[3], PaLM[4], CodeGen[5], and more.
Recently, I have carefully studied the paper[1] on RoPE and derived its formulas. I would like to share them here in the hope of helping readers understand this clever idea.
This article mainly consists of three parts, including an introduction to the underlying principles, visual illustrations, and an analysis of the RoPE code in the Llama model.
The Transformer model owes its remarkable performance to the essential Attention mechanism, which calculates the attention weights between each token in the input sequence.
Let’s assume a sequence has N tokens. The embeddings of the m-th token is xm, and the embeddings of the n-th token is xn.
Without adding position information to the word embeddings, we can transform them into queries qm, keys kn, and values vn as shown in equation (1):
The queries and keys are then used to compute the attention weights, while the output is computed as the weighted sum over the values, as shown in equation (2):
We discovered that when positional information is not included, the attention weight a(m, n) between tokens xm and xn remains constant… Read the full blog for free on Medium.
Join thousands of data leaders on the AI newsletter. Join over 80,000 subscribers and keep up to date with the latest developments in AI. From research to projects and ideas. If you are building an AI startup, an AI-related product, or a service, we invite you to consider becoming a sponsor.
Published via Towards AI