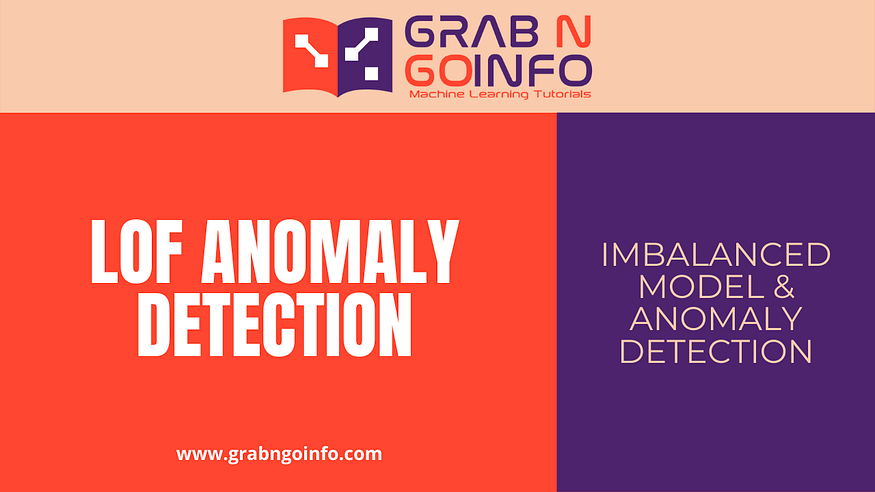
Local Outlier Factor (LOF) For Anomaly Detection
Last Updated on July 26, 2023 by Editorial Team
Author(s): Amy @GrabNGoInfo
Originally published on Towards AI.
LOF for novelty and anomaly detection
This member-only story is on us. Upgrade to access all of Medium.
Image Owned by GrabNGoInfo.com
Local Outlier Factor (LOF) is an unsupervised model for outlier detection. It compares the local density of each data point with its neighbors and identifies the data points with a lower density as anomalies or outliers.
In this tutorial, we will talk about
What’s the difference between novelty detection and outlier detection?When to use novelty detection vs. outlier detection?How to use Local Outlier Factor (LOF) for novelty detection?How to use Local Outlier Factor (LOF) for anomaly or outlier detection?
Resources for this post:
Video for this tutorial on YouTubePython code… Read the full blog for free on Medium.
Join thousands of data leaders on the AI newsletter. Join over 80,000 subscribers and keep up to date with the latest developments in AI. From research to projects and ideas. If you are building an AI startup, an AI-related product, or a service, we invite you to consider becoming a sponsor.
Published via Towards AI