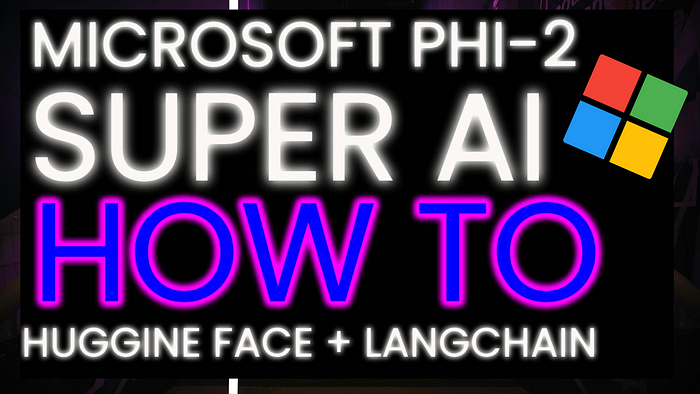
Microsoft PHI-2 + Huggine Face + Langchain = Super Tiny Chatbot
Last Updated on December 30, 2023 by Editorial Team
Author(s): Gao Dalie (高達烈)
Originally published on Towards AI.
Today, Microsoft Research released the latest version of the small language model (SLM) Phi-2, which has only 2.7 billion sets of parameters.
So, In this Post, we will learn what Microsoft Phi-2 is, Why Phi-2 is so small, and how to use Microsoft PHI-2, Huggine Face, and Langchain to create a super Chatbot
It is only about 38% the size of the most anticipated Meta Llama 2–7B (7 billion sets of parameters).
but its performance is said to be comparable to that of Meta Llama 2–7B (7 billion sets of parameters). Comparable to Llama 2–7B and Mistral-7B!
I highly recommend you read this article to the end is a game changer in your chatbot that will realize the power of Microsoft PHI-2!
If you like this topic and you want to support me:
Clap my article 50 times; that will really help me out.U+1F44FFollow me on Medium and subscribe to get my latest articleU+1FAF6Follow me on my Twitter to get a FREE friend link for this article and other information about data, AI and AutomationU+1F52Dphi-2
Microsoft Phi-2 SLM is trained using “textbook-quality” data, which includes synthetic datasets, general knowledge, theory of mind, daily activities, and more.
Microsoft’s Phi-2 can also solve complex mathematical equations and physics problems. On… Read the full blog for free on Medium.
Join thousands of data leaders on the AI newsletter. Join over 80,000 subscribers and keep up to date with the latest developments in AI. From research to projects and ideas. If you are building an AI startup, an AI-related product, or a service, we invite you to consider becoming a sponsor.
Published via Towards AI