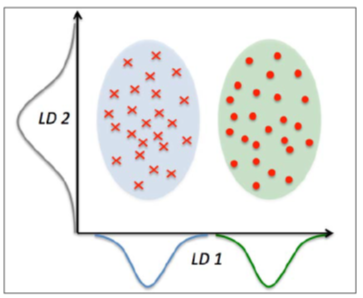
Machine Learning: Dimensionality Reduction via Linear Discriminant Analysis
Last Updated on July 25, 2023 by Editorial Team
Author(s): Benjamin Obi Tayo Ph.D.
Originally published on Towards AI.
A machine learning algorithm (such as classification, clustering or regression) uses a training dataset to determine weight factors that can be applied to unseen data for predictive purposes. Before implementing a machine learning algorithm, it is necessary to select only relevant features in the training dataset. The process of transforming a dataset in order to select only relevant features necessary for training is called dimensionality reduction. Dimensionality reduction is important because of three main reasons:
Prevents Overfitting: A high-dimensional dataset having too many features can sometimes lead to overfitting (model captures both real and random effects).Simplicity: An over-complex model having too… Read the full blog for free on Medium.
Join thousands of data leaders on the AI newsletter. Join over 80,000 subscribers and keep up to date with the latest developments in AI. From research to projects and ideas. If you are building an AI startup, an AI-related product, or a service, we invite you to consider becoming a sponsor.
Published via Towards AI