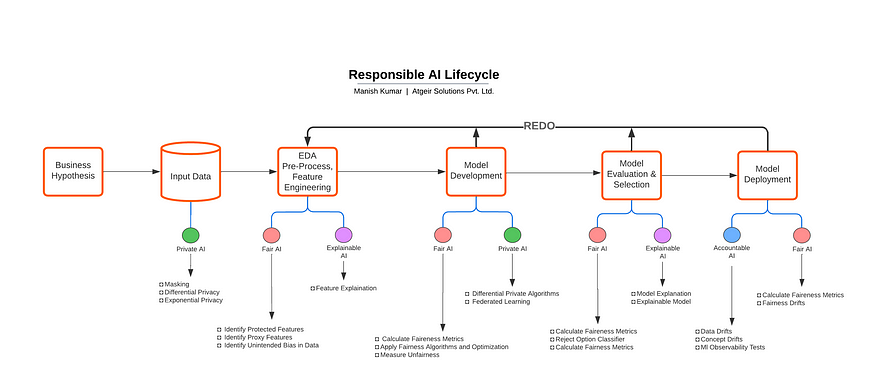
Ethical AI Guardrails for Using Large Language Models (LLMs) in Clinical Trials
Last Updated on June 28, 2023 by Editorial Team
Author(s): manish kumar
Originally published on Towards AI.
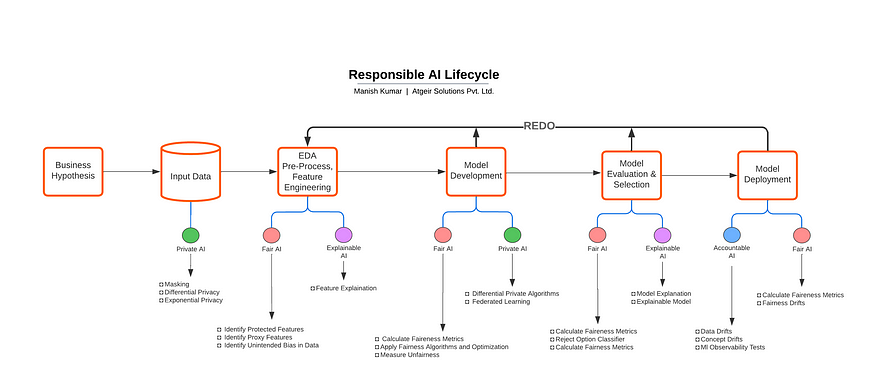
Large Language Models (LLMs) are being used at an unprecedented rate in healthcare, which can fuel innovation in clinical trials. These powerful models, which can understand and generate human-like language output, are used to improve research design, patient recruitment, data analysis, and much more. However, we must follow the principles of Ethical AI as we decide how to use its great potential. By using Ethical AI techniques, we can ensure that including LLMs in clinical trials guarantees the values of objectivity, transparency, accountability, and respect for human rights.
Using LLMs for Clinical Trials
LLMs improve clinical trials. By carefully studying recent trial data and medical literature, LLMs can foresee and identify impediments and inefficiencies that potentially compromise trial quality. These tools must be used morally and for people. Clinical trials that address health inequities can benefit from LLMs. LLMs can identify underrepresented groups to improve clinical trial recruitment efforts. Diversifying patients for clinical trials is crucial. LLM improves clinical trial efficiency and helps make patient-beneficial decisions by examining data.
Risks of Using LLMs For Clinical Trials
Because of their extensive data training, LLMs ignore data biases. Misinterpretations happen. These biases make LLMs prone to misinterpreting complex medical information and drawing poor conclusions. If not addressed, it can impact clinical trial outcomes. Because of their complexity, LLMs are usually called “black boxes,” making it difficult to comprehend how they obtain their conclusions. A lack of transparency makes it difficult to analyze outcomes and conceal biases and errors. Because of its powerfulness, human researchers may depend too heavily on LLMs rather than their knowledge and experience. Whatever their powers, LLMs should only be used to support human decision-making. Healthcare AI is rapidly expanding, but regulations are behind. This might pose compliance and legal concerns. LLMs can be used ethically in clinical research if the risks are addressed. These mitigation techniques include extensive testing, audits, security, and human monitoring.
Data Privacy
Clinical research employing LLMs requires data privacy. Assume that LLMs rely on patient health records for trial participants. For privacy, the LLM should pseudonymize or anonymize this data. Synthetic data, duplicating real-world data’s statistical aspects without revealing patient-specific information, might further increase privacy. Pharmaceutical companies investigating new drugs use synthetic data. The corporation trains the LLM with electronically generated data that resembles the target patient group instead of actual data. AI can be used without compromising patient privacy. Data privacy requires informed consent, which LLMs can help with. LLMs can use legislation and medical research to create patient-friendly information sheets and permission forms.
Transparency
LLMs are similar to “black boxes” in that they are difficult for outsiders to comprehend because of how intricate they are. But it’s crucial that everything be evident when these models are applied in clinical investigations. Consider using an LLM to analyze the clinical study’s data and determine, for instance, the efficacy of some specific measure. Researchers should be able to comprehend how the LLM arrived at its judgment and identify any potential biases if the explanation is transparent and simple for others to comprehend.
Immutable Storage and Data Processing
Using LLMs in clinical trials relies heavily on secure data processing and storage. For instance, clinical trial data can be protected in a Write Once, Read Many (WORM) state utilizing a technology akin to immutable storage. As a result, data saved for a set amount of time cannot be changed or removed. Also, a time-based retention strategy may be established for all patient data, trial findings, and LLM-produced analysis in a multi-year clinical trial testing some medications. This eliminates any chance of data tampering or unintentional change and guarantees that the data is consistent and secure throughout the trial. Similarly, a legal hold policy might be introduced for delicate trials under regulatory review, enabling the data to be stored unaltered until the legal issues are resolved. Such secure storage techniques guarantee data integrity, promoting confidence in using LLMs in clinical studies.
Cybersecurity Checks
When LLMs are used in clinical studies, cyber security is critical. Because clinical trial data is private, it’s essential to have robust cybersecurity measures in place. For LLM infrastructure to be safe, there must be regular penetration testing, system updates with the latest security changes, and a proactive way to find and deal with possible threats. Imagine that a biopharmaceutical business is using an LLM to look at the results of a global clinical study. The company must protect the LLM and the vast network of systems it connects to. This is done with the help of regular vulnerability checks, strong firewalls, safe cloud storage systems, and attack detection systems.
Training
Human capital is an essential part of security. Whether they are researchers, data scientists, or IT staff, people who work with LLMs should know the best ways to keep data secure and private. This goes beyond just technical training; they should also learn about the ethical issues when AI is used in clinical studies. Let’s say that a clinical research team uses an LLM to look through patient health information to find people interested in participating in a clinical study. The team should know how to use the LLM well and understand the rules about patient privacy, consent, and how to handle data. This ensures they can use the tool fairly and decently and reduces the chance of data breaches.
Conclusion
In conclusion, the strategy taken in medical research may be significantly changed by using Large Language Models (LLMs) in clinical trials. However, this must be done with a firm commitment to responsibility, security, responsible use, data privacy, and transparency. Synthetic data and digital twins can be used as practical tools to make clinical trials safer and more effective as we learn to use LLMs to their maximum potential.
For instance, by examining the reactions of digital twins, which are effectively virtual patient models, LLMs can predict the effectiveness of a new cardiac medicine. As a result, clinical studies are more effective, and there is a lower chance that patients may have unfavorable outcomes. If we keep in mind these guidelines and are open to learning new ones, we may use LLMs to improve the standard of clinical trials and, therefore, the results of health research investigations.
Join thousands of data leaders on the AI newsletter. Join over 80,000 subscribers and keep up to date with the latest developments in AI. From research to projects and ideas. If you are building an AI startup, an AI-related product, or a service, we invite you to consider becoming a sponsor.
Published via Towards AI