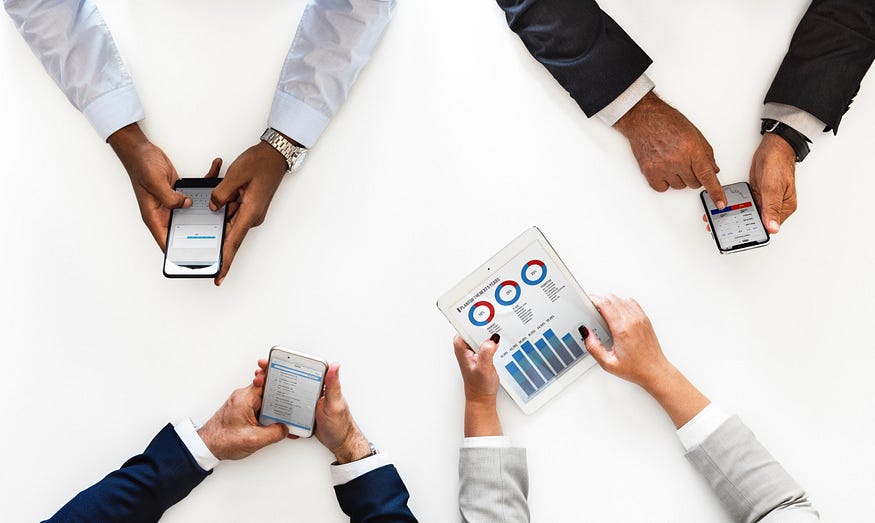
Application of Synthetic Minority Over-sampling Technique (SMOTe) for Imbalanced Datasets
Last Updated on July 25, 2023 by Editorial Team
Author(s): Navoneel Chakrabarty
Originally published on Towards AI.
In Data Science, imbalanced datasets are no surprises. If the datasets intended for classification problems like Sentiment Analysis, Medical Imaging or other problems related to Discrete Predictive Analytics (for example-Flight Delay Prediction) have an unequal number of instances (samples or data points) for different classes, then those datasets are said to be imbalanced. This means that there is an imbalance between the classes in the dataset due to a large difference between the number of instances belonging to each class. The class having comparatively fewer instances than the other is known to be a minority with respect to the class… Read the full blog for free on Medium.
Join thousands of data leaders on the AI newsletter. Join over 80,000 subscribers and keep up to date with the latest developments in AI. From research to projects and ideas. If you are building an AI startup, an AI-related product, or a service, we invite you to consider becoming a sponsor.
Published via Towards AI