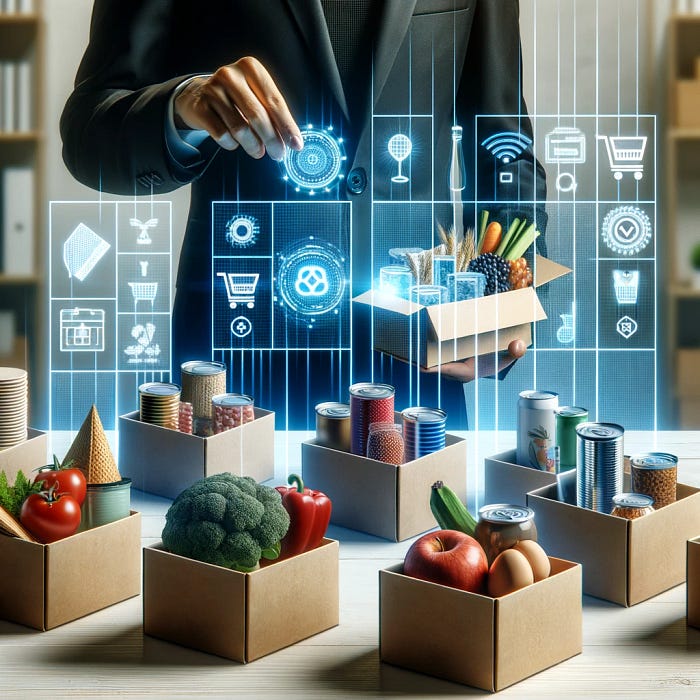
Machine Learning and Operations Research for Optimizing Assortment Size
Last Updated on February 22, 2024 by Editorial Team
Author(s): Alexandre Warembourg
Originally published on Towards AI.
Explore my journey in tackling a multi-objective constrained optimization problem to achieve assortment reduction without losing customers and revenue using genetic algorithms and machine learning.
Source : Dall-E
In retail, assortment planning is critical. It involves balancing a diverse range of products, from budget to premium, either focused on own-brand products or niche for a specific type of customer. Our scenario was the first, and our goal was to refine an overly broad product offering to improve operational efficiency and customer experience.
Auchan is known for its gigantic product range, and when you enter an Auchan hypermarket, you’re guaranteed to find the product you’re looking for, given the sheer number of references per unit of need. A few years ago, this tendency was a major asset, but today it’s less and less the case. Having too many products leads to a dispersal of sales, increases costs and can confuse the customer experience.
To define this gigantic offer, we defined a reference number that can be broken down into groups from A1 to A10 for each unit of need, which we'll call box size. Each store can position itself on a box size per need unit, and depending on the size, it will have access to a wider or narrower range of products.
These sizes were defined by industry experts many years ago, and have not been challenged very often…. Read the full blog for free on Medium.
Join thousands of data leaders on the AI newsletter. Join over 80,000 subscribers and keep up to date with the latest developments in AI. From research to projects and ideas. If you are building an AI startup, an AI-related product, or a service, we invite you to consider becoming a sponsor.
Published via Towards AI