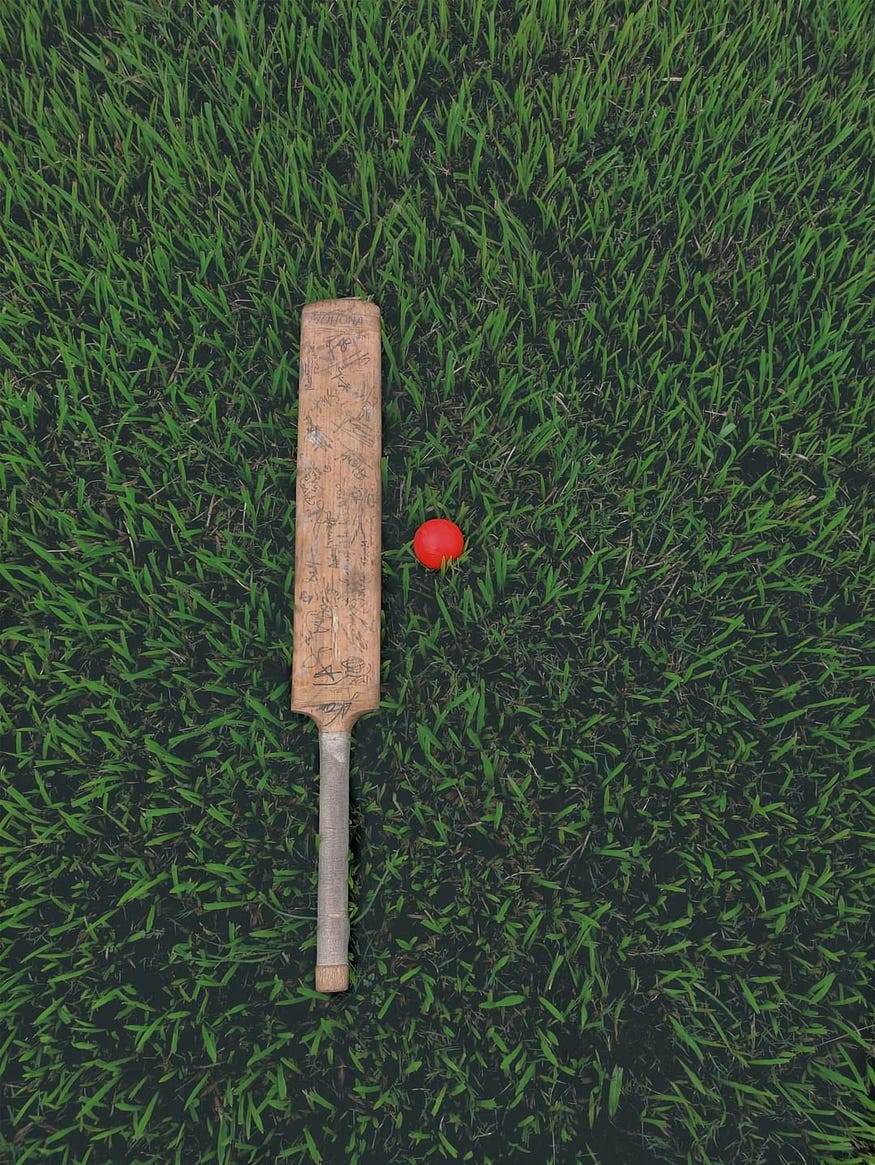
MoneyBalling Cricket: Predicting Centuries — Base Model
Last Updated on July 26, 2023 by Editorial Team
Author(s): Arslan Shahid
Originally published on Towards AI.
Data preparation & binary classification modeling of centuries in cricket
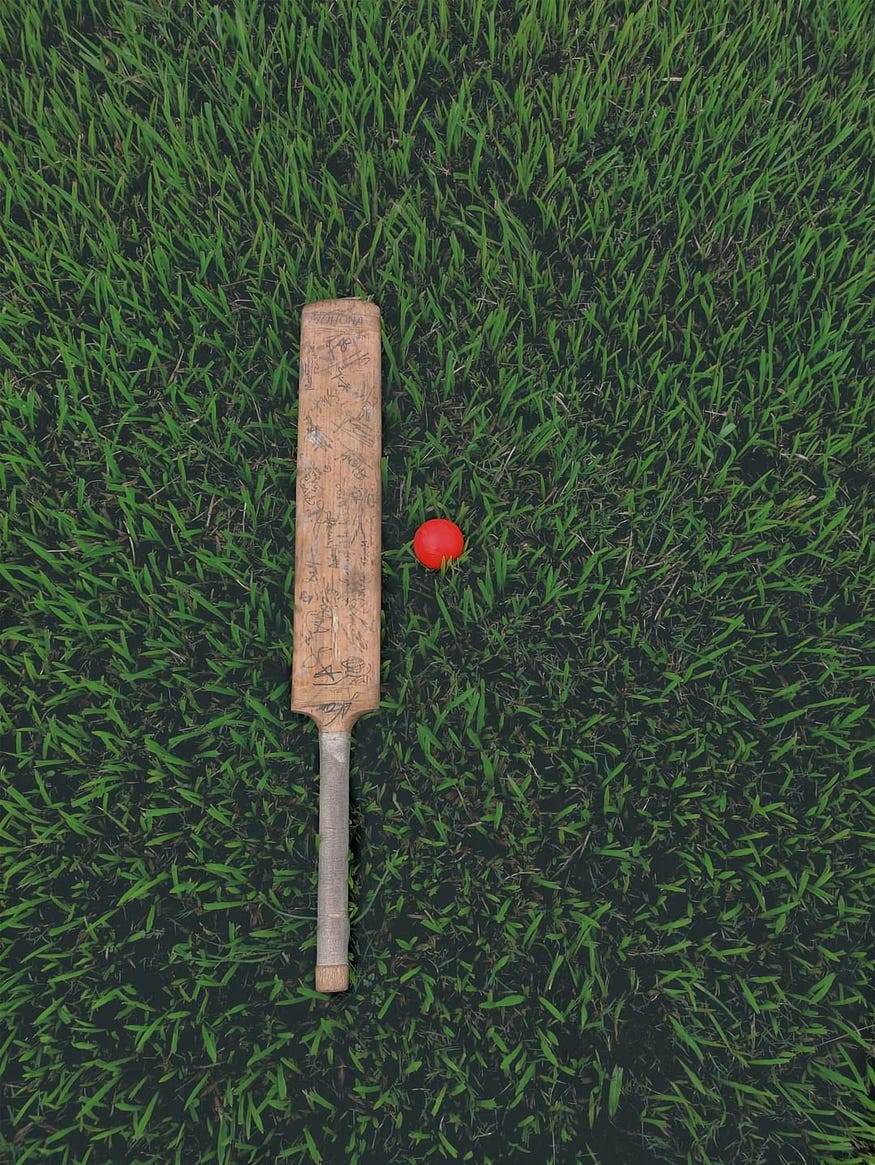
Centuries are a celebrated event in cricket, usually resulting in match-winning innings by the batsman. As a statistics enthusiast, it felt like a great problem to model because it is not only immensely interesting, the novelty of the problem did make it challenging. This piece explains the reasoning behind how I prepared the data, what model I used, and the evaluation criteria.
Data
Before starting, a bit of information about the data source and verification.
- Data Source: All the data has been sourced from cricsheet.org. They offer ball-by-ball data of ODIs, T20s, and Test matches. I do not own the data, but cribsheet data is available under the Open Data Commons Attribution License. Everyone is free to use, build and redistribute the data with proper attribution under this license. Read about the license here.
- Data Verification: The founder of cricsheet does a good job of verifying the data source with minimal errors. I verified the data using aggregates and compared them with aggregates available at major cricketing sites such as ESPNcricinfo.
- Data Dimensions & time: The dataset contains 2050 ODI matches, starting from 2004–01–03 to 2022–07–07. It contains almost all major male ODIs played during the period. The dataset contains 1,087,793 balls played, & 35,357 batsman knocks & 4,002 innings.
In a previous post, I did a probabilistic analysis of centuries, a key finding was that unconditioned on anything else, the empirically estimated probability of a batsman knock resulting in a century is only 3.16%. This is important because when modeling a classification problem, class prevalence is probably the most crucial factor in determining the efficacy of your model(s). A low-class prevalence usually means that model performance on metrics like accuracy, precision, recall & f1 score will be low. In simple words, it will be hard to predict centuries if you predict a century at the start of the match, you need to overcome this somehow to get any meaningful results.
Money Balling Cricket — Probability of 100 using repeated conditioning
Probabilistic analysis of Scoring ≥ 100 runs in cricket
towardsdatascience.com
Simplifying the problem
Any model trained with data sampled at the start of the match is unlikely to have predictive power, to mitigate this problem, I needed to simplify the problem. If one were to predict the centuries at the halfway point where the models are predictive, like the moment the batsman reaches a threshold of 50–55 runs, they would get much better results.
Another simplification to make is to exclude data points when a century is not ‘possible’; when the balls remaining don’t permit a century without free hits, and for the second innings when the total required to win is lower than the required runs for a century. This would reduce noise in our data. The last simplification is to exclude teams that do not play Test cricket, I did this because these teams have very small samples compared to major teams.
All of this simplification might seem like cheating, but when you are modeling, do the most simple thing you can do first, then remove some of these restrictions to get a series of more ‘complete’ models.
Data Preparation

The original dataset extracted from cricsheet is ball by ball, the way I designed our model, it will take in snapshots of the batsman’s innings the moment they cross 50 runs. The following steps were taken to prepare it for modeling.
- Identifying rows or instances in the matches where a batsman in one innings crossed the 50 runs threshold for the first time.
- These were then passed through a series of filters.
- The first filter checks that both batting and bowling teams are Test-playing teams.
- The second filter ensures that a century is still possible, considering the number of balls remaining.
- The third filter deletes rows where the batsman could not complete their century if the target score doesn’t allow for one, it only applies to the 2nd innings.
At these snapshots, all historical data (up to the current ball of the current match) of the batsman was aggregated. For the batsman, their historic average against the team they are batting against, and for the bowling team, the historical average of the economy (runs per ball), runs per wicket, etc, against the same team were computed. In case of no history, they were imputed with an overall team historical KPIs, before the innings in question. Also, partnership statistics like total runs by the current partnership & partner’s score were added to the dataset.
These historic KPIs were important for making an informative model but including them poses the risk of target leakage. It happens when you train your algorithm on a dataset that includes information that would not be available at the time of prediction. In our case, it can happen if I include in the training dataset the historic KPIs of a match that happens after a match in the test dataset. To prevent this from happening, the data had to be sorted by time & balls played in the match, with only the first 80% of the data included for training, and the rest 20% was put into the test dataset.

Base Model
For my initial model, I choose a Binary Logit model a.k.a Logistic Regression. The model was chosen for the following reasons:
- Interpretability: Complicated modeling techniques such as neural networks will likely perform better on performance metrics, but this comes at the cost of interpretability. Logistic Regression is easy to interpret, which often gives insights into how our independent variables impact the dependent variables.
- Debugging: In most modeling exercises, you often have to debug your data & model. The outliers and confounding effects are easier to identify in a simple model. Which can help you clean your data or do better feature engineering.

Note: hist economy is the historic economy of the bowling team overall against the batting team, up to that match & hist Avg is the historic average of the batsman against the same bowling Team. Both were imputed with historic overall average/economy if there is no history between the batting team & bowling team.
Have a data science problem, and need an expert to solve it? Consider hiring me!
Model Evaluation
The model was evaluated on a test dataset on a series of metrics, which change as you change the decision boundary of your model. Logit Models predict the probability of the event happening, it is up to the modeler to pick the cut-off threshold used to classify an event as a century or not a century.
By default, that decision boundary is set to 0.5 ( a predicted probability of >0.5 is 1 and else 0), but that fails in most cases when there is a huge class imbalance. One class is more prevalent than the other, so to overcome this, you change the decision boundary.
Below are the intuitive explanations of all the metrics used to evaluate our model:
Precision:
Formula — (True_positive)/(True_positive + False_positive).
Intuition: Maximizing precision means that you do not include any false positives in your predictions, you only include those predictions which are extremely likely to be true positives. This intuitively means that your predictions will include many false negatives but fewer or no false positives.
Recall:
Formula — (True_positive)/(True_positive + False_negative).
Intuition: Maximizing recall means that you capture all those instances which are true positives, and do not include any false negatives. This means you will tolerate false positives but not false negatives. There is a tradeoff between precision & recall.
F1 score:
Formula — 2*(precision*recall)/(precision + recall).
Intuition: The F1 score is the harmonic mean of precision & recall. Maximizing the f1 score is where you reach a ‘mid-point’ between precision & recall. Where you scrutinize false negatives & false positives equally.
F-beta score :
Formula — (1+Beta²)(precision*recall)/((Beta²)precision + recall).
Intuition: Similar to F1 score the F-beta score also tries to reach a ‘consensus-point’ between precision & recall but the beta value skews the consensus in favor of either precision or recall. A beta value greater than 1 means a skew in favor of recall & a beta value lower than 1 means a skew towards precision.

The curve above shows how model metrics change if the decision boundary of the model is changed. Which metric to maximize is purely a question of how you want to use the model. For example, in sports betting if you want to bet big on a player making a century, you would like to be very sure that your prediction is a true positive, so you might want to optimize the model on the precision or f beta score with a lower than 1 beta value.
In most cases, one would want to choose a point that penalizes both false positives & false negatives equally, so the f1 score makes the most sense. F1 score is maximized at an 18% threshold, the model has an f1 score of 48%, an accuracy of 60%, and a recall of 70%. This means the model captured 70% of all true positives, but the precision of 38% is low. Any improvements to this base model should be more precise with its prediction!
These were the metrics used to find the optimal decision boundary, now, let us evaluate the model as a whole. For that, I used the Area under the curve (AUC) of the receiver operating characteristic (ROC) curve.

Intuition (ROC): The ROC curve shows the relationship between True positive rate (TPR) & false positive rate(FPR). The true positive rate is the same as recall. The ROC tells how the two change, remember to capture more true positives, you have to also tolerate more false positives. The origin line is where both TPR & FPR are equal, a model which has TPR = FPR for all decision boundaries is purely making random predictions.
Intuition (AUC): The Area under the curve is a metric of the overall performance of our model. The AUC ranges from 0 to 1, with 0 having no predictive power, 1 meaning perfect predictive power & 0.5 meaning purely random predictive power (no better than flipping a coin).
The origin line forms a triangle with the x-axis, with length & height = 1. This means that it has an AUC of 0.5 (Area of triangle = 0.5 * height * length). Our model’s ROC curve has an AUC of 0.653, which implies it is much better than random!
Improvement in our model can be made in two ways, use more sophisticated algorithms or try predicting centuries at a threshold other than 50–55 runs!
Thank you for reading! I will explore the different scoring thresholds and different, more complicated models in my next post on the topic. Stay Tuned!
Want to read more about statistical modeling in cricket, please do check these out:
Money Balling Cricket — Statistically evaluating a Match
Evaluating player performances using descriptive statistics
medium.com
Money Balling Cricket: Averaging Babar Azam’s runs
One of the key elements in the movie Moneyball(2011) is that Billy Beane (Brad Pitt) and Peter Brand (Jonah Hill)…
arslanshahid-1997.medium.com
Or maybe you want something different:
Lies, Big Lies, and Data Science?
I’m sure that with all the hype surrounding data science, machine learning, and artificial intelligence, you’ve been…
medium.com
Please do follow me on Medium, Twitter, and Linkedin. Don’t forget to click the email icon so that you can receive an email of my posts.
Join thousands of data leaders on the AI newsletter. Join over 80,000 subscribers and keep up to date with the latest developments in AI. From research to projects and ideas. If you are building an AI startup, an AI-related product, or a service, we invite you to consider becoming a sponsor.
Published via Towards AI