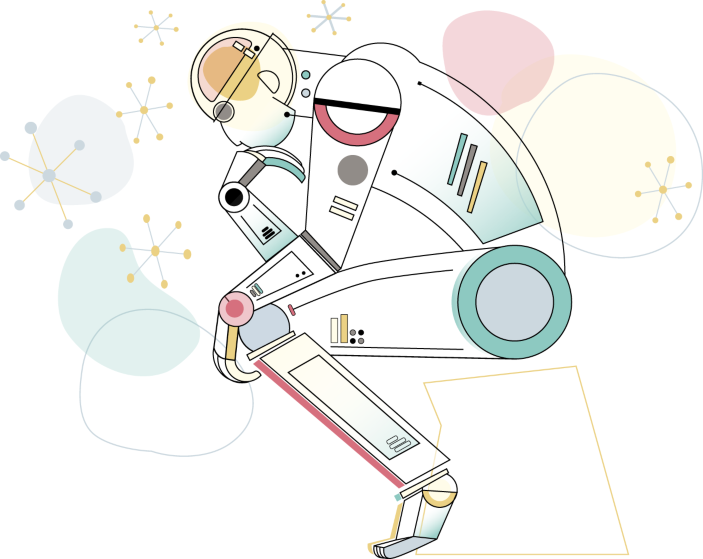
Not So Common Machine Learning Examples That Challenge Your Knowledge
Last Updated on October 12, 2021 by Editorial Team
Author(s): Gaurav Sharma
Machine Learning
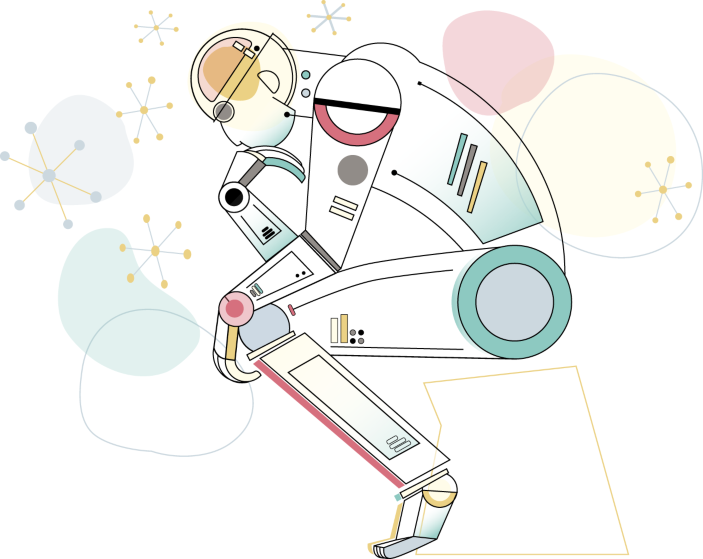
Machine Learning refers to the process through which a computer learns and changes its operations based on patterns identified in vast quantities of data. When we think about machine learning, we think of a few well-known instances. For example, the way Amazon recommends products is remarkably similar to Google searches you’ve done. Machine learning’s reach is far broader than what we are familiar with and observes in our daily lives.
Because machine learning is such a young science, the boundaries of its applicability are continuously being pushed outside. Virtual personal assistants were once the stuff of fantasies, but now they can be found in every other home. While some instances are obvious, there are several more ways that machine learning is affecting our lives that you may not have considered.
PREDICTION AND DETECTION OF EARTHQUAKES
Machine learning has recently been used to detect seismic waves and evaluate patterns from a million hand-labeled seismograms using data collected and machine learning. More than twice as many earthquakes are detected by the algorithm as by scientists. Scientists will be able to identify earthquakes in near real-time when a significant quantity of data has been collected and trends established.
Because of the nature of machine learning, it may soon be feasible to forecast earthquakes ahead of time. This has a huge influence on disaster management forces, healthcare, firemen, and other emergency responders’ preparation and readiness.
WHALES IN THE OCEAN IDENTIFICATION
Underwater noise pollution poses a serious hazard to marine mammals like whales. Machine learning has recently been discovered to be capable of processing acoustic data and reliably recognizing whales. Participants were invited to submit their best machine learning algorithm for detecting whale cry sounds from audio recordings in a competition hosted by Marinexplore and Cornell University. Cargo carriers, agents, and other interested parties may utilize the information to plan maritime routes and avoid crashes if a whale is discovered. Whales’ noise footprints would be reduced if ships were rerouted away from them.
https://www.youtube.com/watch?v=tSVHDzOZ_-Q
The beluga whales of Cook Inlet are endangered, and machine learning might be the key to saving them. Underwater sounds disturb marine life, and the resulting disturbances can cause behavioral changes and physiological damage to the creatures. Scientists can plan the recovery of this whale population by using algorithms to precisely and quickly understand the patterns of the whales.
USE OF SOCIAL MEDIA TO DETERMINE MENTAL HEALTH
A 9-year-old competition led to the notion that psychopathic traits may be detected in people based on their language patterns and social conduct, both of which can be analyzed using Twitter. A survey of Reddit users was recently performed in a similar fashion. The algorithm learned to identify persons with mental health issues by accumulating information from user postings on specific forums.
Since the epidemic made in-person contacts impossible, social media usage has exploded in recent years. In addition to increased social media usage, the ongoing epidemic has resulted in a rise in the number of individuals suffering from poor mental health. It would be extremely beneficial to develop a method for identifying persons who are dealing with their mental health. Researchers who use machine learning to evaluate indications of mental health disorders may share their findings with businesses, organizations, and people.
There are existing applications that converse in a human-like manner to assist users to overcome loneliness and enhance mental health, such as Replika. Imagine a platform that detects the most vulnerable people based on their social media posts and directs them to applications like Replika for early intervention.
INDUSTRY OF BEAUTY
Machine learning is frequently mentioned as a way for consumers to evaluate how goods might look on their skin from the comfort of their own homes. Producers, too, utilize this technology to evaluate goods while they are still in the creation stage.
https://www.youtube.com/watch?v=2tmJw1_XPA0
Machine learning is increasingly being used in the beauty sector to produce goods that are more cost-effective and timely. The same technology is now being used to ‘objectively’ rank people based on their attractiveness. Apps that conduct this rating require the individual to submit a photo of their face without makeup and compare it to other people’s photos. Aspects such as symmetry, wrinkles, dark circles, and facial imperfections are all taken into account. This is an unsettling thought, not just because many individuals are sensitive about their looks, but also because of the mental health consequences of body dysmorphia and other associated disorders.
The issue arises: why do we need to rank people based on their physical appearance? If we truly think that “beauty is in the eyes of the beholder,” then an algorithm that compares and analyzes people and their appearance is just unsettling. It goes against the concept that beauty is subjective and might have disastrous consequences for people’s self-perceptions.
PREDICTION OF SPORTS INJURY
In the sports sector, a large amount of data is accessible for analyzing player performance, success rate, and other statistics. One of the most intriguing applications of machine learning in this sector is how it may be used by sports teams and coaches to anticipate injuries. The system analyzes muscle movement data and detects recurring patterns, allowing teams and coaches to be notified when the default patterns are disrupted.
This information is useful in the player’s preventative care. The sports team will save millions of dollars in medical expenditures, missed income, and recovery costs as a result of this. However, while every professional athlete is aware of the danger of injury, having an algorithm predict that you would be injured within this time frame (assuming high accuracy) might have a severe impact on the player’s mental health and performance.
MACHINE LEARNING IN THE FUTURE
Data is necessary for machine learning models to work. Even the most performant algorithms can be rendered worthless without a foundation of high-quality training data. Indeed, when machine learning models are trained on insufficient, incorrect, or irrelevant data in the early stages, they might be handicapped. When it comes to training data for machine learning, the old adage “garbage in, garbage out” still holds true.
This blog demonstrates that machine learning is both dynamic and adaptable. Its applications span industries and even time. Machine learning is becoming increasingly important as a result of the huge amounts of data accessible and the fact that ML successfully collects, analyzes, and interprets this data. In parallel, the challenges that the IT sector may solve using machine learning are becoming increasingly concerning. It’s critical that we use our heads to find innovative, relevant, and ethical applications for the amazing instrument that is machine learning.
Not So Common Machine Learning Examples That Challenge Your Knowledge was originally published in Towards AI on Medium, where people are continuing the conversation by highlighting and responding to this story.
Published via Towards AI