Why Are We So Afraid of AI?
Last Updated on August 18, 2021 by Editorial Team
Author(s): Seppo Keronen
Opinion
We humans have become the dominant species on “our” planet. We are afraid of anything or anyone, too powerful or different from ourselves — How alien is the emerging Artificial General Intelligence (AGI), and how could we possibly avoid or overcome such a threat?
On the other hand, our instinctive drive for dominance means that we humans act without due regard for consequences. We build nuclear weapons, we exhaust and pollute our environment, we exploit the weaknesses of others. We all know this, and this makes us afraid of our own shadow! Is AGI going to be a stronger and even scarier version of ourselves?
A third perspective — Human societies are in the process of becoming more peaceful, fair, just, and tolerant. Will intelligent machines help us keep the peace and will they free us from trudgery and poverty?
Finally — Artificial intelligence technology has been over-hyped ever since the early years of computers when they were called “electronic brains”. Are we just going through yet one more hype cycle that we can ignore?
In order to make sense of where we stand, let’s first reflect on ourselves as intelligent biological machines. Given this benchmark, we can better understand and compare ourselves with machine intelligence that may emerge. Doing this we arrive at startling conclusions!
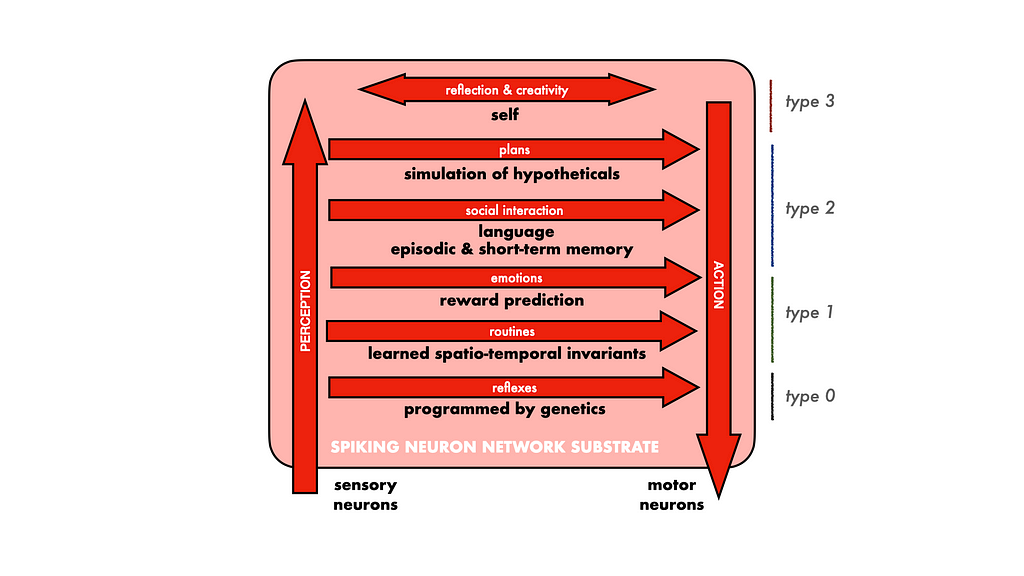
Human Intelligence
As illustrated in figure 1, we perceive our environment (including the state of our own body) via sensory neurons, and we act via motor neurons. Our intelligence, or the lack of it, is what happens as the perceived sensory signals are processed, by our biological neural network, to produce more or less impressive current and future actions.
We may distinguish levels of processing/thinking that have developed over millions of years of evolution. Evolution is a largely conservative process that seems to have retained the more ancient structures as new ones have been overlaid. Starting from the most ancient layer and working up to where we are now:
Level 0 — reflexive responses hard-wired during development by our genes. These neural circuits are only minimally, if at all, modified functionally during our individual lifetime.
Level 1 — highly parallel learning and exploitation of spatial-temporal patterns that have predictive power for survival. The learning signal here is mismatch of expectation and subsequent ground truth. There are also value signals here that motivate us and that we experience as emotions.
Level 2 — attention focused processing of expressions composed of symbols (signifiers) denoting entities, qualities, quantities, feelings, relationships and processes. This makes the human species a powerful super-organism able to transmit information between individuals across space and time.
Level 3 — we are able to compose (imagine) hypothetical entities, situations, processes and other intensions without the corresponding external data (extension). This enables self-referential thinking and makes us into individually powerful engines of creation.
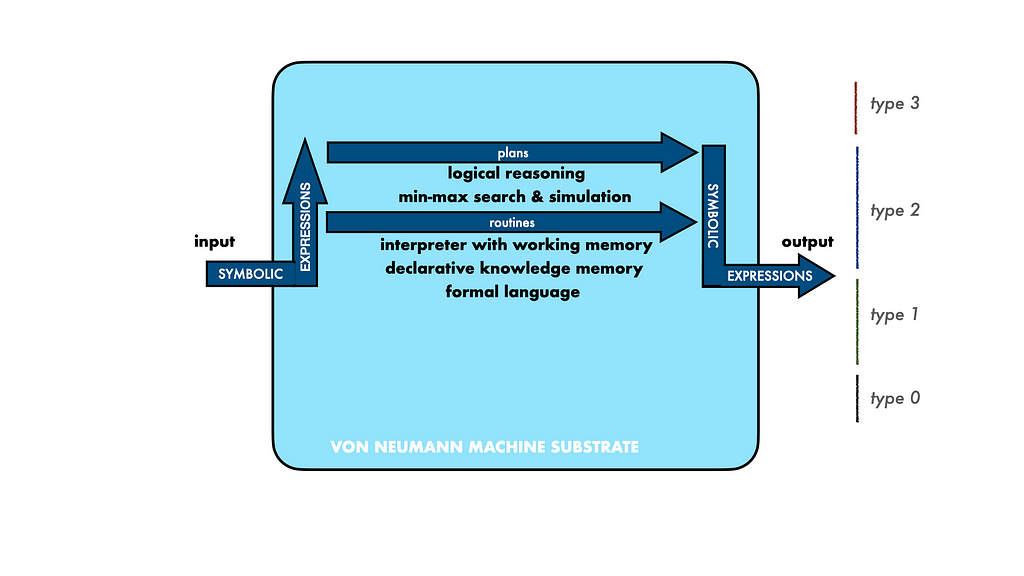
Symbolic AI (Expert Systems, GOFAI)
Boolean logic circuits and memory registers, from which computers are made, are ideal for arithmetic calculations and other symbolic operations. Symbolic AI is the practice of programming this computational substrate to emulate the operations of rule-following symbol rewriting systems, such as predicate calculus and production systems. Notably, the reverse, human computational substrate (networks of stochastic neurons and limited short-term memory) emulates symbolic computation very poorly.
During the second half of the 20th century, the term AI was used to refer to the practice of representing “knowledge” in the form of input symbolic expressions and processing these to produce output symbolic expressions. This Symbolic (good old-fashioned AI) tradition is illustrated in figure 2. Here we also illustrate it corresponding with the type 2 thinking of the benchmark model.
It was found that symbolic AI suffers from critical shortcomings, which eventually lead to a decade or so of depressed funding of research (AI winter) around the turn of the century. The main shortcomings identified were:
Grounding — A symbolic language works as a useful tool when its symbols refer to elements of the world of interest, and its statements correspond with actual and potential states of that world. Symbolic AI does not provide representations for such referent entities, processes and semantic interpretation.
Learning — Instead of learning, symbolic AI relies on a repository of knowledge formulated as statements in a formal language. The scarce availability of experts to encode and verify such knowledge bases is referred to as the knowledge acquisition bottleneck.
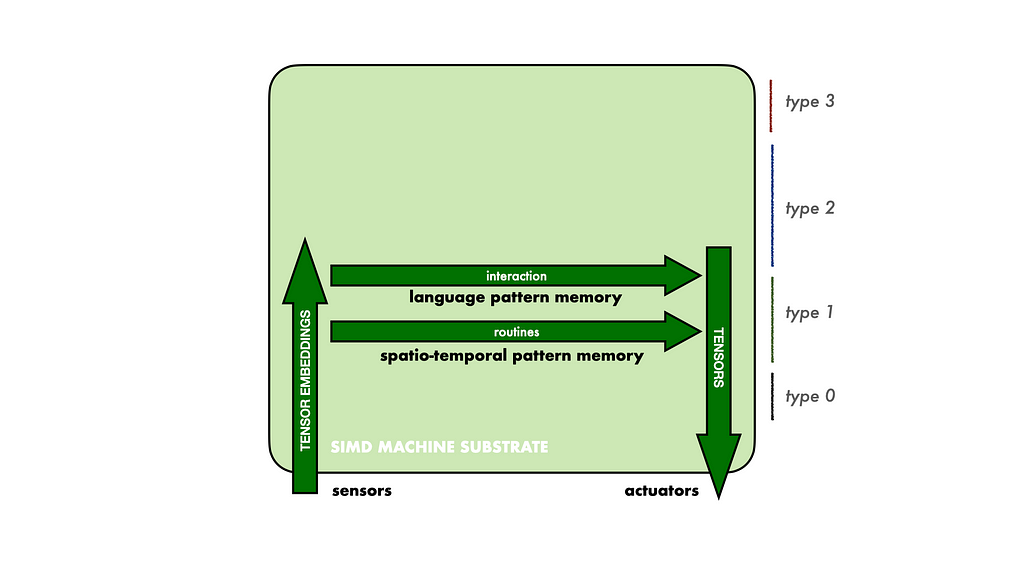
Connectionist AI (Neural Networks, DNNs)
Connectionist AI is n alternative to the above symbolic tradition. Here we program (or otherwise arrange) the circuits and memory cells of computer hardware to emulate the operation of networks of neuron-like elements. This approach has been pursued by dedicated researchers since the 1940s. Notably, a traditional von Neumann computer is not well suited to efficiently emulate a biological nervous system. and many simplifications and alternatives are pursued.
The terms deep learning and deep neural networks (DNNs) are often used to refer to the current incarnation of the connectionist AI paradigm. The emphasis here is on three key principles:
Representation — Multidimensional vectors of numbers (tensors) are used to represent all data and state.
Processing — A large number of layers of non-linear neuron-like elements are deployed to increase modeling power.
Learning — Statistical learning of patterns in data using a back propagation algorithm is used to embed long-term memory (weights) in the static state of the network.
Figure 3 illustrates DNN capabilities relative to our benchmark architecture. Relative to symbolic AI, DNNs are a bottom-up, type 1 technology that can accept multimodal sensory input (images, audio, touch, etc.) and drive actuators (speakers, displays, motion etc.) without complex encoding and decoding.
The application of DNNs is enabling a new industrial revolution combining mechanical power and computation to produce adaptive machines able to perform tasks previously requiring human, and even super-human effort. The large economic opportunities are driving research and development to overcome the limitations of current models and practices. The core, immediate problems, and associated opportunities are:
Language — Language and other symbolic expressions are used to carry information about a domain of reference. Current connectionist architectures are not able to exploit this critical power.
Episodic Memory — Current models do not have adequate mechanisms to recognize and represent non-stationarities in data.
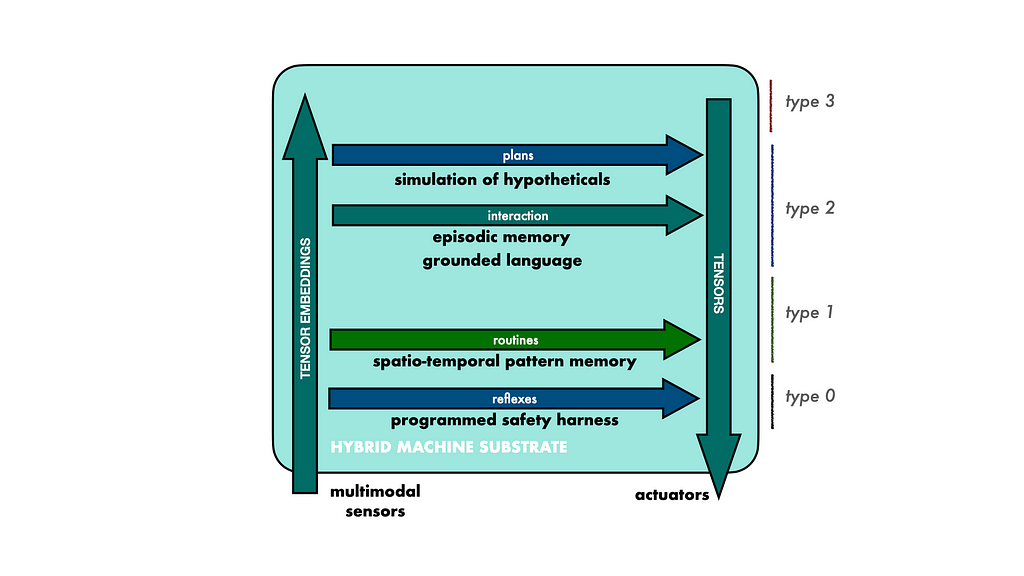
Artificial General Intelligence (AGI)
Assuming that the reference schema of Figure 1 is meaningful, it is clear that we are far from replicating human-like minds. That said, the endeavor to understand and engineer intelligence is part of our journey to discover who/what we humans are and what options we have for the future.
Figure 4 illustrates a feasible, prospective architecture for autonomous machines, spanning type 0, type 1 and type 2 characteristics. We can look forward to safe, autonomous machines that can learn new skills by reading instructions, perform useful work and even amuse and entertain us.
Safety — We will incorporate policy to ensure safe decisions and fail-safe behavior. Even after some notable failures, experience with fly-by-wire aircraft safety indicates that such programmed safety harnesses are feasible.
Language — Providing machines with grounded referential natural language is probably the most exciting, high return challenge that we are ready to take on right now.
Episodic Memory — Grounded symbolic representations of data will enable more efficient and expressive memory structures to deal with the complexity of the world, with partial models of the world constantly subject to improvement.
Hypotheticals — Even machines need to hallucinate possible worlds and delay “gratification” to make plans for coherent orchestration of action.
Autonomous machines can be used to either set us free or to enslave and destroy us. These machines themselves have no agenda, the agenda is determined by us. If we are afraid, we are afraid of ourselves!
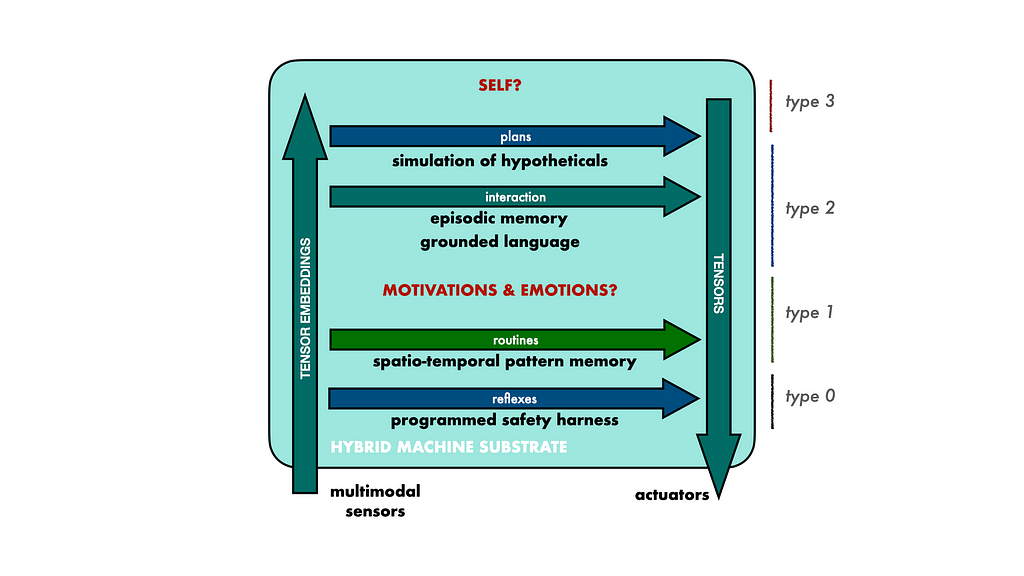
The “dark matter and dark energy of intelligence”, illustrated in figure 5, still remain relative to the human benchmark of figure 1. What hides behind the words “self”, “motivations” and “emotions” and the “feelings”, “qualia”, “awareness” and “consciousness” associated with them? We don’t seem to even have the required concepts to answer such questions. Perhaps together with the AIs, we will discover some answers?
Why Are We So Afraid of AI? was originally published in Towards AI on Medium, where people are continuing the conversation by highlighting and responding to this story.
Published via Towards AI