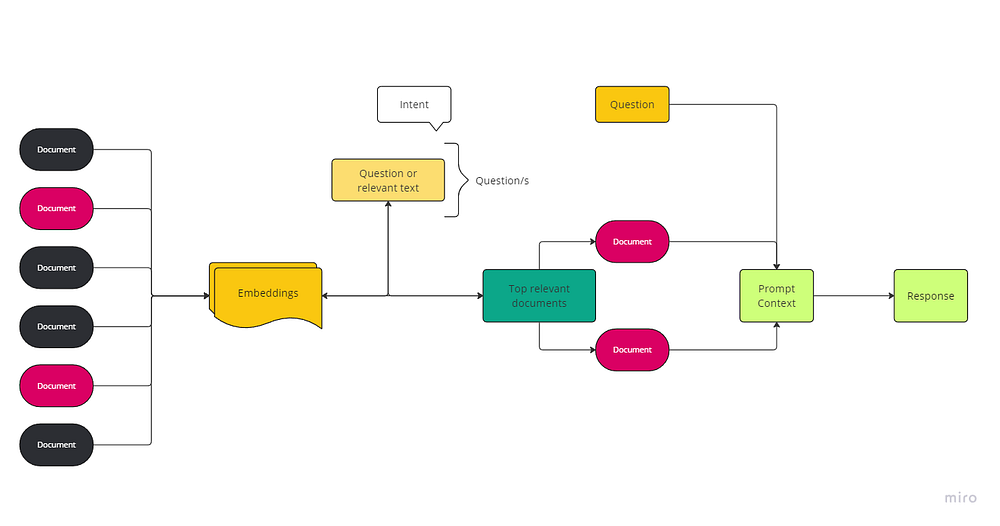
RAG in Production: Chunking Decisions
Last Updated on April 7, 2024 by Editorial Team
Author(s): Dr. Mandar Karhade, MD. PhD.
Originally published on Towards AI.
Prototype to Production; All about chunking strategies and the decision process to avoid failures
Different domains and types of queries require different chunking strategies. A flexible chunking approach allows the RAG system to adapt to various domains and information needs, maximizing its effectiveness across different applications. Like you, I am not here to build another generic chatbot, but I want us to be able to create tools for niche domains. That's where the value of RAG systems for most businesses is. Thats where the challenge is. So, let's dive in.
Note: There are some sections in italics — Make sure to read those ones if you are short on time
Retrieval-Augmented Generation (RAG) is a type implementation of the AI system where the AI output is potentiated / augmented by providing it with the specific precursors of the information. This process is called as retrieval in-short for retrieval of the relevant information. In case of the generative models the retrieval is generally followed by Generation. The core idea behind RAG is to augment the language generation process with external knowledge by dynamically retrieving relevant documents or data during the generation phase. This approach allows the model to produce more accurate, informative, and contextually relevant responses, especially in domains requiring specific, detailed information.
In this article, we will… Read the full blog for free on Medium.
Join thousands of data leaders on the AI newsletter. Join over 80,000 subscribers and keep up to date with the latest developments in AI. From research to projects and ideas. If you are building an AI startup, an AI-related product, or a service, we invite you to consider becoming a sponsor.
Published via Towards AI