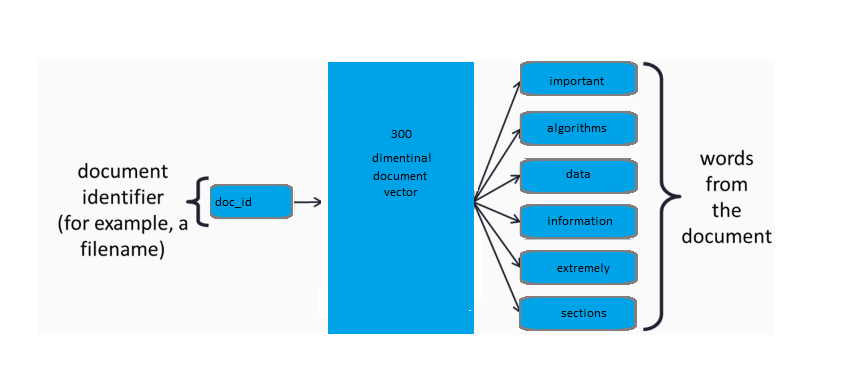
An Intuitive Introduction to Document Vector(Doc2Vec)
Last Updated on July 20, 2023 by Editorial Team
Author(s): Manish Nayak
Originally published on Towards AI.
Intro to Doc2Vec U+007C Towards AI
Introduction
Doc2Vec is an extension of Word2vec that encodes entire documents as opposed to individual words. You can read about Word2Vec in my previous post. Doc2Vec vectors represent the theme or overall meaning of a document. In this case, a document is a sentence, a paragraph, an article, or an essay, etc.
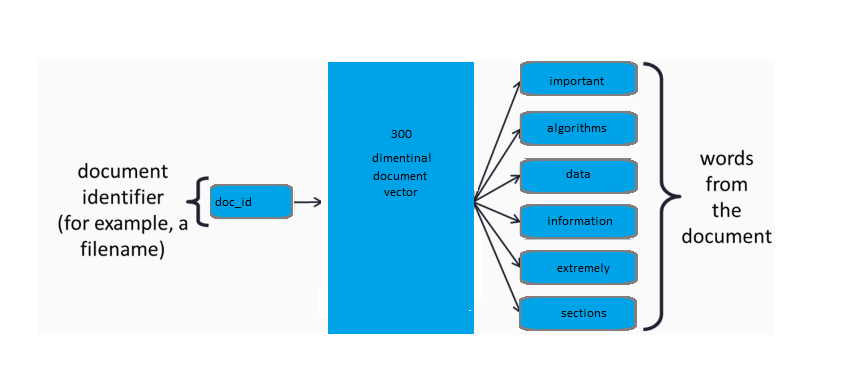
In Doc2Vec, the name of the document, like file name or file ID will be the input, and the sliding window of the words from the document is the output.
Similar to Word2vec, there are two primary training methods, Distributed Memory Model Of Paragraph Vectors (PV-DM) and Paragraph Vector With A Distributed Bag Of Words (PVDBOW).
They are variations of the CBOW Models and Skip Gram Models, which use to train Word2Vec, it as extending the idea of context to paragraphs by adding a label or a document-ID.
- The Distributed-Memory Model closely resembles the CBOW model of Word2vec. This model tries to predict a target word given its surrounding context words with the addition of a paragraph ID.
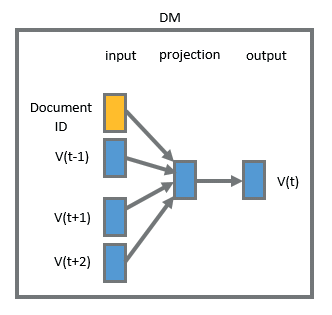
- The Distributed Bag-Of-Words Model based on the Word2vec skip-gram model, with one exception instead of using the target word as the input, It takes the document ID as the input and tries to predict randomly sampled words from the document.
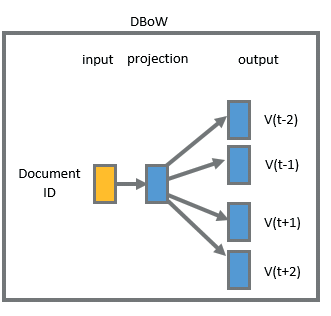
The input filename is not much important. Output words are essential and need to keep track of them as they came from the same document. All these words are connected to that filename.
Doc2Vec model can predict the document’s words based on its filename, the Doc2Vec model knows which words go together in a document.
The content of documents usually about one thing, for example, many different positive words are used in positive reviews, and many negative words are used in negative reviews.
After training, we can have a new document for which we need to find its document vector. Doc2Vec uses the word similarities learned during training to construct a vector that will predict the words in the new document.
Once we get that new document vector, we can compare this document vector with other document vectors and find which document vectors from the past are the most similar.
So, Doc2Vec can use to find similar documents. This will help us to find the positive and negative reviews because, in general, document with the positive reviews will have related vector and document with the negative reviews will have related vector.
Accompanied jupyter notebook for this post can be found on Github.
Conclusion
We know the importance of vector representation of documents, for example, documents clustering or classification tasks, the documents need to be represented as a vector.
I hope this article helped you to get an understanding of Doc2Vec. It will also provide inside of Doc2Vec representation of documents, and its uses.
Join thousands of data leaders on the AI newsletter. Join over 80,000 subscribers and keep up to date with the latest developments in AI. From research to projects and ideas. If you are building an AI startup, an AI-related product, or a service, we invite you to consider becoming a sponsor.
Published via Towards AI